Everlaw AI Assistant Is Transforming How Orrick Handles Litigation Discovery
Innovative approach increases speed, accuracy, and value for clients
by Petra Pasternak
Key Takeaways
In a live IP case, Orrick ran Everlaw Coding Suggestions on ~10,000 documents.
EverlawAI Assistant was more accurate than human reviewers
Orrick estimated more than 50% in doc review cost savings
The advent of generative AI in ediscovery has been hailed as a fundamental and transformative change.
Like many legal professionals, Orrick attorney Cal Yeaman needed to see it in action to understand how this new technology fit into existing work.
What level of nuanced analysis was the algorithm capable of? How did it compare to human review? Could it be as consistent as a predictive coding tool?
Orrick, a tech-forward firm with a deep history of innovation, tested and then deployed EverlawAI Assistant Coding Suggestions on a real-world IP matter. The new Everlaw GenAI feature leverages the reasoning capabilities of large language models to expedite the review determinations process.
Coding Suggestions classifies documents for relevance through human prompts, and performs at accuracy levels that matched or exceeded humans in an earlier series of Everlaw customer tests.
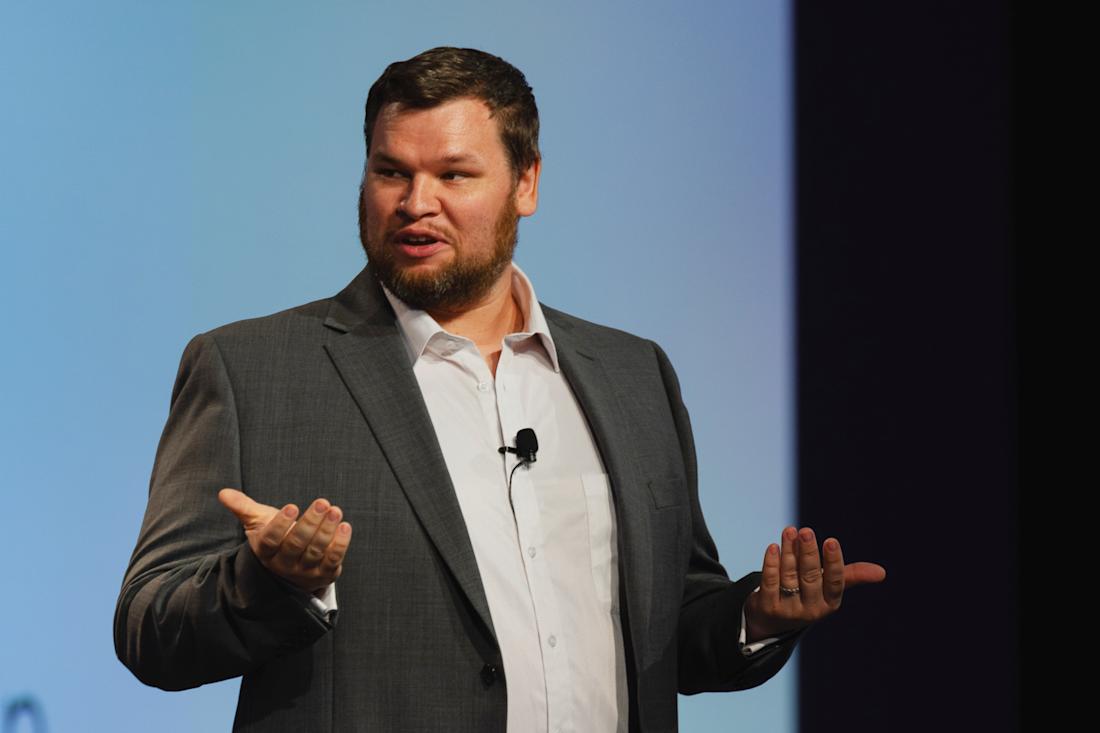
Finessing a New Hybrid Workflow
The Orrick team tested and deployed this new technology on approximately 10,000 documents in an IP case. They started with a small sample subset to test and refine the prompts for Coding Suggestions before applying the prompts to the bigger document set.
To evaluate the effectiveness of the AI, Orrick created a hybrid workflow using both Coding Suggestions and Predictive Coding. The idea, Yeaman said, was to use the generative AI tool to speed up the sampling process for predictive coding, and predictive coding would help validate the accuracy of the generative AI output.
"Everlaw Coding Suggestions reduced the cost of document review by more than 50%."
Combining Predictive Coding With Coding Suggestions
While both Predictive Coding and Coding Suggestions are used to classify documents, they are based on different technologies:
Predictive Coding learns from human reviewers as they code documents to continuously evaluate patterns and generate predictions about the likelihood of relevance for a given document.
Coding Suggestions, on the other hand, relies on individual prompts from humans, which provide the necessary context of the case and goals of the review.
While Predictive Coding models evolve with a review, refining Coding Suggestion prompts involves testing and iteration as instructions are improved and results validated.
AI Performance Results: A Very Powerful Tool
As the review process unfolded, Yeaman’s initial skepticism turned into enthusiasm. It became clear that Coding Suggestions scored high on both accuracy and consistency.
The Predictive Coding tool was used to help validate the accuracy of the generative AI via statistical sampling. It also helped prioritize documents that would most likely require a human quality control review.
The results impressed him, Yeaman said during a keynote presentation at Everlaw Summit ’24.
"AI coding suggestions were more accurate than human review."
Coding Suggestions showed a level of accuracy and consistency that would help reduce attorney time on document review, free up more time to develop case strategy and reduce client costs.
“I was surprised to find that the generative AI coding suggestions were more accurate than human review by a statistically significant margin,” Yeaman said. Of the documents identified by GenAI as not-relevant, the human reviewers only reversed the decision on a single document.
That may not always be the case, he noted. AI performance will vary from matter to matter, he said, depending on the criteria of a particular review, the nature of the case, the types of data, and the underlying subject matter.
Running the numbers, Yeaman estimated that the new AI-powered review process reduced cost of document review by more than 50%.
The new tool features also accelerated Orrick’s ability to identify key documents quickly, empowering the team to know the evidence and their case faster than ever before, Yeaman said.
“It’s a very powerful tool.”
Orrick’s Tips and Takeaways for Success
“I highly encourage people using these tools to do so in a small sample set first,” Yeaman said. “If you get into the documents a little bit early, look around and identify specific documents to iterate on so that you know what you're working with.”
He also cautioned to recognize that the value of a tool is in its application.
“Coding Suggestions cannot be used on many of the same types of documents that the Predictive Coding struggles to process. You need the right kind of case for the right kind of tool – the more linear the analysis the more the LLM is favored over a human team. The more complex the subject matter the more the LLM is favored over the human team.”
Yeaman saw testing Coding Suggestions on a document-rich and complex IP case as the next natural step in Orrick’s efforts to deliver better client service at greater value through innovation.
“What's critical is knowing the market and testing tools that can add value and speed to our services – and to work with trusted technology partners.”
If you’re interested in learning more about generative AI, or want to see how EverlawAI Assistant can improve your workflows, request a demo today.

Petra is a writer and editor focused on the ways that technology makes the work of legal professionals better and more productive. Before Everlaw, Petra covered the business of law as a reporter for ALM and worked for two Am Law 100 firms.