GenAI Document Review Surpasses Human Performance on Real World Litigation Data
New White Paper Evaluates Everlaw AI Assistant Coding Suggestions
A new Everlaw report reveals that generative AI tools can achieve remarkable accuracy in document coding for litigation.
In a test of EverlawAI Assistant Coding Suggestions against four real-world, human-reviewed datasets, the tool was able to successfully classify documents as responsive or nonresponsive to specific prompts, achieving accuracy levels comparable to human reviewers generally. In one instance, where direct comparison was available, the AI's performance surpassed first-level human performance on recall by 36%.
These promising results indicate that much of the first-pass review typically handled by legal professionals can be achieved with the help of GenAI tools to alleviate the burdens of manual workflows. The implications for ediscovery workflows and resource allocation in document review – one of the most costly and time-consuming phases of litigation – are considerable.
About the Experiment
The experiment pitted Coding Suggestions, part of the EverlawAI Assistant suite of generative GenAI tools, against first-level human reviewers, across four ediscovery data sets comprising a total of 7,737 documents. These were all part of active civil litigation matters involving a broad range of formats, including PDFs, emails, spreadsheets, calendar files, and general text files.
EverlawAI Assistant Coding Suggestions works by leveraging large language models pretrained on enormous data sets to apply user-directed coding criteria to a set of documents. User coding criteria include descriptions of the case, the corresponding coding category, and the individual codes. For example, these may be as broad as potential attorney-client privilege, or as specific as individual elements and sub-elements of a claim.
In this test, based on interviews with customers and provided review protocols, Everlaw was able to create initial prompts for Coding Suggestions that were case specific.
Coding Suggestions then rated the documents as “yes” suggestions, meaning the document directly matched the criteria, “soft yes,” “no,” meaning there was no relevance to the criteria, or “soft no,” indicating the document was weakly relevant.
Results Show Strong Performance in Recall and Precision
Comparing the suggested codes against first-pass review allowed the team to determine performance based on both precision and recall.
Precision is a measure of how many documents are actually relevant out of the documents predicted to be relevant – or how well the predictions match reality.
Recall is a measure of how many relevant documents the model identified as a percentage of the total number of documents that are actually relevant.
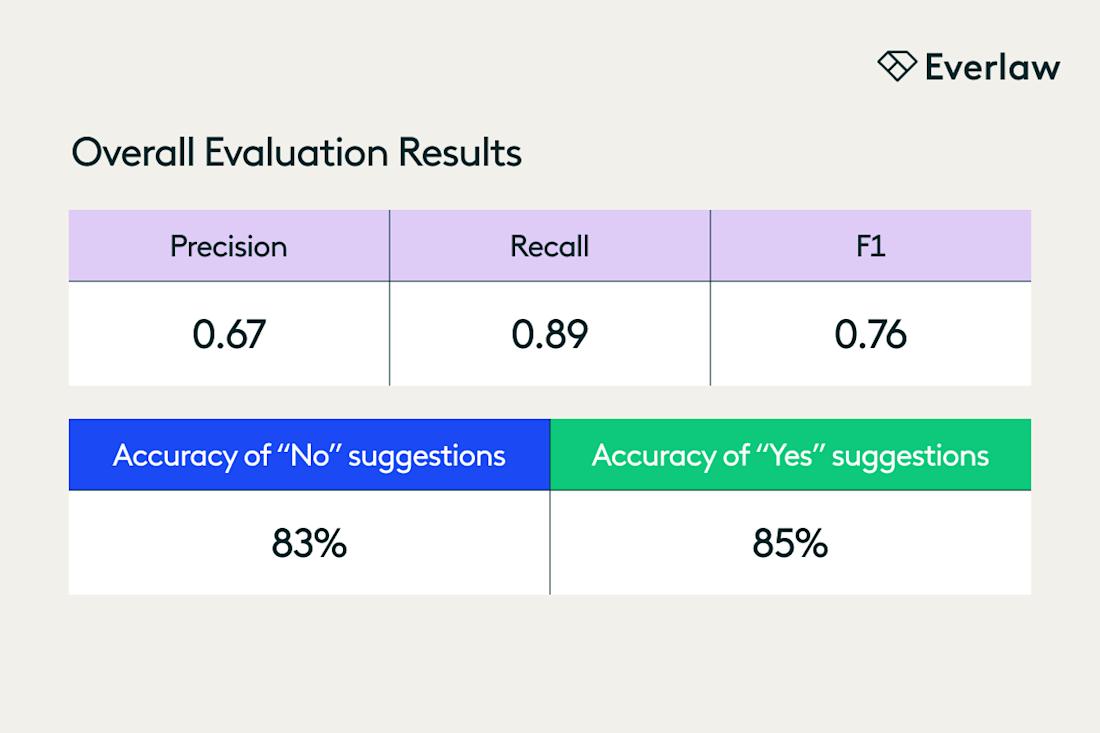
When aggregated across all four data sets at the “yes” and “soft yes” cutoffs, Coding Suggestions delivered a precision score of 0.67 and a recall score of 0.89.
These results are well in the range of human manual review performance, where first-level human review teams achieve recall scores ranging from 0.25 to 0.8 and precision scores between 0.05 and 0.89.
What’s more, in one data set where second-pass review allowed direct comparison between Coding Suggestions and the first-level human reviewers, Coding Suggestions outperformed humans on recall by 36%, achieving 0.82 compared to reviewers’ 0.6.
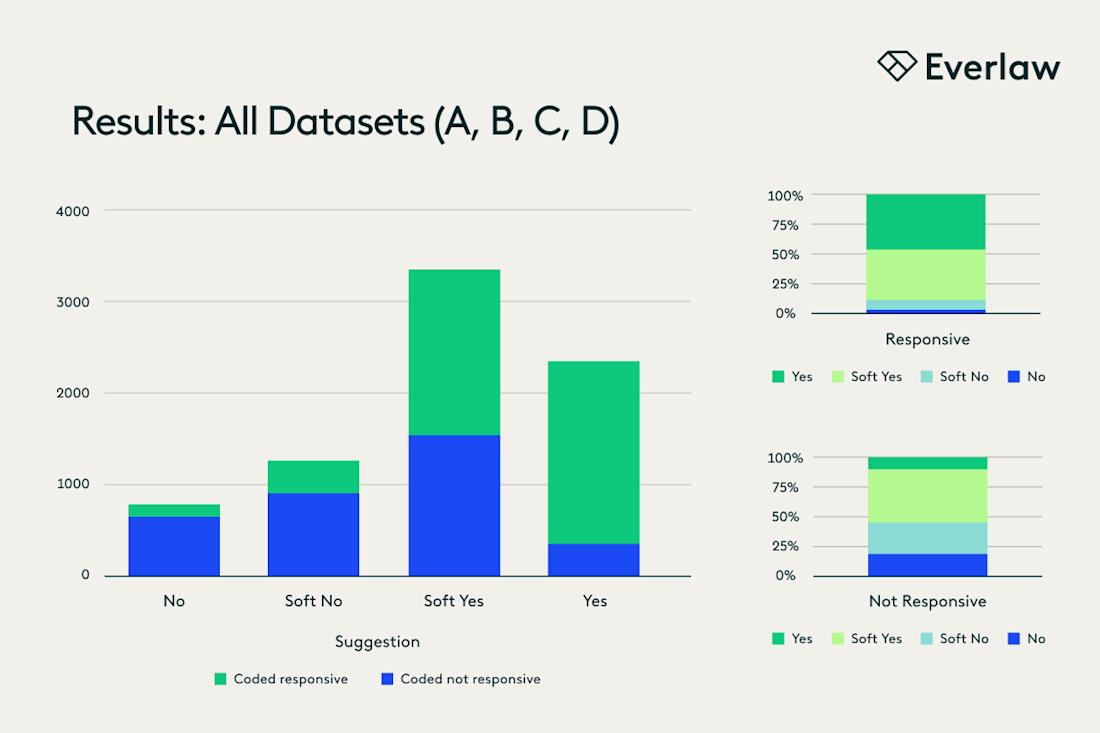
Learn More in the Full Report
Everlaw’s tests of Coding Suggestions are preliminary but promising, suggesting that these tools can perform at or above first-level human review. Of course, there are caveats, but what these initial tests show is that legal professionals can reasonably rely on Coding Suggestions performance to help prioritize and classify documents.
What’s more, the results legal professionals can achieve in the real world can be higher with prompt refinement and iteration.
To see the full report, including a detailed breakdown of performance, download your copy today.