Generative AI Demystified
Everlaw's AJ Shankar on Today's Breakthrough Moment
by Casey Sullivan
When ChatGPT 3.5 was released into the world just under a year ago, the potential impact of this new generative AI application was almost immediately understood by legal professionals. A true leap forward in GenAI technology, tools like ChatGPT, Anthropic’s Claude, Google’s Bard, LLama, and more, can perform tasks that would have seemed unthinkable before – draft a sonnet, write a speech, pass the bar.
Hundreds of law firms quickly convened task forces to understand the new technology, its implications, and how best to apply it. It was a reaction that truly underscored the potential impact of GenAI, a mobilization in response to technology that was truly unprecedented in the history of the legal profession.
Or, as Everlaw founder and CEO AJ Shankar noted as he began his keynote on generative AI at Everlaw Summit, “GenAI is here to stay. This is the real deal.”
But even as legal professionals move to take advantage of generative AI – enthusiastically, but responsibly — to many, the technology itself remains an enigma.
During his keynote presentation, AJ sought to demystify the workings of GenAI, how we got to the technology we have today, and where GenAI-powered legal tools may be headed in the future. By better understanding generative AI, practitioners can not only better appreciate its strengths and weaknesses, but also understand how best to apply GenAI tools, explain them to their clients and stakeholders, and, ultimately, get the most value out of this technology.
watch aj's keynote presentation from everlaw summit below:
From Machine Learning to Generative AI
To understand how we arrived at today’s GenAI tools, we need to start with machine learning. The most widely applied form of artificial intelligence, machine learning technology utilizes computer models that can adapt and evolve (or “learn”) without being explicitly programmed. They are adaptable, mutable models, as opposed to traditional, static algorithms.
The difference between a machine learning model and a standard algorithm is the difference between a calculator and a sports coach, AJ analogized. A calculator is fixed. It doesn’t learn as you feed more calculations into it and its approach to multiplication will never alter. “That’s exactly what you want,” he noted, “for a calculator!”
But that rigid approach is not always the best approach. Take the example of a coach. “A coach who has exactly the same playbook at the end of the year as she did at the beginning is probably not going to do as well as one who adapts her playbook as the season goes on,” AJ said. “Machine learning systems are like a coach who learns from experience. They perform better by adapting when given more data.”
“GenAI is here to stay. This is the real deal.”
Machine learning allows for similar growth by relying on statistical models rather than static code, models that can evolve to reflect the latest learnings. “The model is what you consult, not the code that created it,” AJ explained. “So get used to thinking about the model rather than the algorithm. There isn’t one.”
Generative AI grew out of these machine learning systems. But unlike some more familiar forms of machine learning, such as predictive coding or concept clustering, generative applications, well, generate. They create new content rather than analyzing existing data. The most relevant of these tools to legal professionals is large language models, or LLMs.

The Evolution of Large Language Models
LLMs can read and write in natural languages, like English. They are what allow GenAI tools to write those sonnets, draft those speeches, and even pass the bar. “This opens up a whole new world of use cases, especially in law,” AJ explained, “since so much work is done in natural language.”
The breakthrough moment in LLMs has a unique history. “LLMs are all neural networks,” he pointed out, “technology that is over 50 years old.”
“Built on graphs with nodes and edges, they’re called neural networks because the structure is similar to our brains, with their neurons and axons.” Within these models, information flows from node to node, with computations happening along the way.
“So get used to thinking about the model, rather than the algorithm. There isn’t one.”
By the 1980s, work on “deep” neural networks had advanced significantly. But they faced formidable obstacles around compute power – the underlying hardware simply wasn’t efficient enough to perform the type of calculations needed – and training data.
As a result, the potential of these technologies was underrealized. That is, until video games changed things.
That’s correct. You can thank games like Doom and Tomb Raider for eventually bringing us GenAI. The explosion in gaming with 3D graphics in the 1990s drove the rapid development of graphics processing units, or GPUs. “GPUs, it turns out, are extremely well suited for training neural networks, much more so than CPUs,” AJ explained.
Suddenly, the limitations on compute power were no longer blocking the growth of neural networks.
Paired with the proliferation of information on the internet, there was now an immense amount of data to train these systems on, and the power to do it.
However, there were still a few hurdles to overcome.
Making the Leap Through Word Embeddings
Several innovations were still needed to get us to the state of generative AI that we see today. “The first major innovation came with inventing a better way to understand the meaning of words in relation to each other,” AJ explained. Past models had treated words in isolation, as individual entities without relationships to each other.
“But that’s not how we as humans think of words,” he said. “The meanings of some words are closer to each other than others.” Happy and glad are more related than happy and mad, cat and dog more than cat and hat, and so on. Understanding those relationships is essential to determining meaning.
This is where the concept of word embeddings becomes pivotal. “This new technique of word embeddings moved from representing words as just the ordering of their letters,” AJ explained, “to a much deeper representation of meaning by encoding each word into hundreds of numbers that essentially encompass what the word means.” And because these are now represented as numbers, the meanings can be treated similar to a mathematical problem. “Take the embedding for ‘king,’ for instance,” AJ demonstrated, “and subtract the embedding for ‘man’ and add the embedding for ‘woman,’ and the result will give you ‘queen.’”
AJ continued, “with embeddings, LLMs can operate on the meanings of words, instead of any particular choice of words themselves. Focusing on meaning allows them to be much more expressive and powerful.”
Understanding Context With Transformers
The second breakthrough dealt with understanding the meaning of the word in the context of the other words around it. “The breakthrough here is called a transformer,” AJ explained, “and it provides a way for an LLM to focus its attention in the right place to understand what a word means.”
Take, for example, two sentences. The first: “The mouse nibbled on the carrot because it was hungry.” The second: “The mouse nibbled on the carrot because it was yummy.” What does “it” refer to here? It’s obvious to us, but would have been terribly confusing to AI of the past. Transformers allow for the consideration of context within a string of words, so that AI can understand that “it” means the mouse in the first sentence and the carrot in the second, just as easily as you or I can.
The Human Key to GenAI
By the 2020s growth in compute power and training data had allowed for rapid development in LLMs. Massive models started to demonstrate truly emergent properties, becoming, for example, far more fluent. From 50 billion parameters in these “earlier” models, sizes have exploded. “It turns out,” AJ noted, “that a certain amount of scale is necessary for intelligence. As soon as researchers saw performance picking up, they invested heavily in scaling up model size further.”
Enter some human fine-tuning, and things really began to take off. “It turns out that putting humans back into the loop makes a huge difference,” AJ illustrated. After standard training, humans provided reinforcement, giving feedback on which responses worked and which fell flat. The scale of this feedback was massive, and essential to the increased fluency that we see today.
But the evolution of these tools is not over. “You should expect even more rapid change from here on out,” AJ promised.
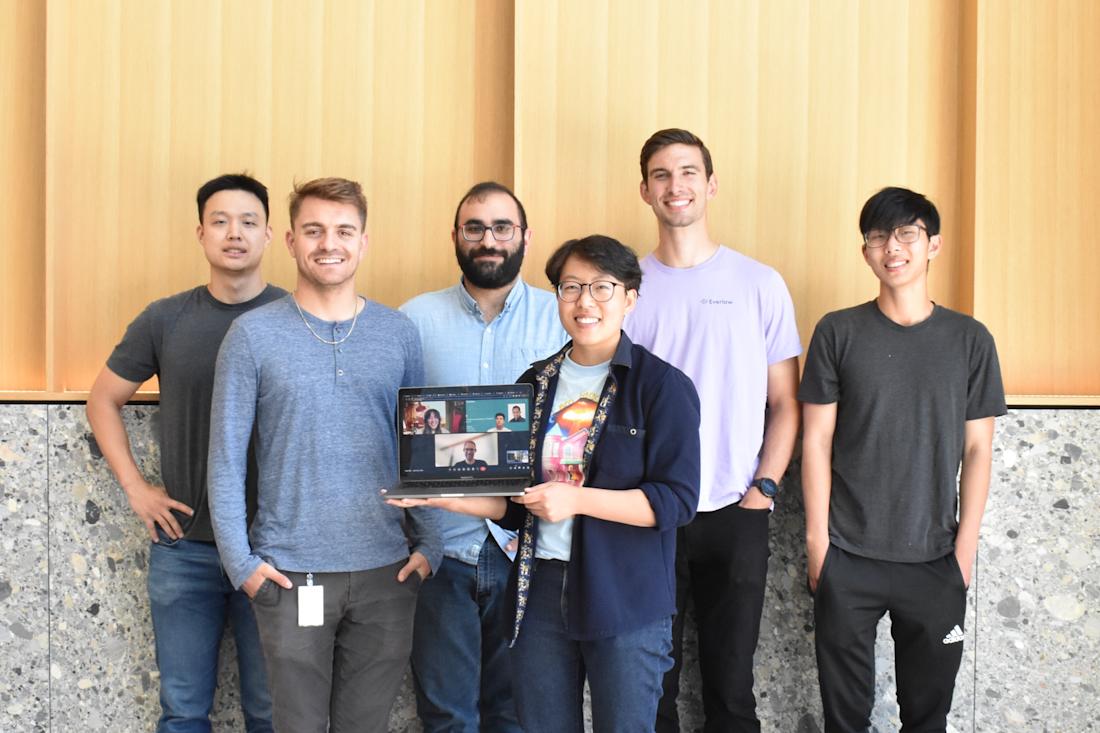
The Role of Training and Inference in GenAI
These are the technological breakthroughs that got us to where we are today. But, AJ asked, “How do these things actually work?”
Through two main aspects: training and inference. Training is how the systems learn, while inference is how they generate content.
“The essence of the training process is actually quite straightforward,” AJ shared. “Feed the LLM a sentence but leave out one word. Then ask it to fill in the blank.” The LLM will rely on word embeddings and transformers to understand the sentence and its neural network to make a guess.
Take the sentence, “The sky is blue.” Drop one of the four words in succession. “___ sky is blue,” for example. The LLM may guess “my,” “the,” or “underwater.” If the guess is good, it moves on. If it isn’t, it will go back and update its parameters to indicate the right answer so that it can make a more likely guess next time. “Do this across tens of billions of sentences and the model quickly learns natural language,” AJ said.
So, how do we get from filling in a blank in a sentence to writing thousands of words independently? This is where inference comes in. “It works in almost the same way: by filling in the blank,” AJ demonstrated.
“But this time, the blank is at the end of the sentence.” The LMM fills in the next word, based on what preceded it, over and over and over. “The…” becomes “The sky…” and “The sky is…” and, finally, “The sky is blue.”
Trained on billions of sentences, the models know what is statistically the most likely word to follow, based on the context.
“You should expect even more rapid change from here on out.”
“It has no understanding of the sky or blueness,” AJ continued. “It is just predicting words. There is no deeper explanation.” But it can do so in a remarkably effective fashion.
“I find intuition is the right metaphor for understanding what is going on here,” AJ said.
“If you think about how you develop intuition, it’s by getting lots of practice with something, and then your brain synthesizes the information so that you can put it to use automatically.” Imagine the chess grandmaster who’s developed great intuition by playing thousands of games, and can thus intuit the best moves in a blitz game without having to rigorously evaluate each position. Similarly, LLMs get practice with a huge swath of human knowledge and expression via their training and can then synthesize that information during inference.
“These LLMs essentially have the world’s best intuition about human knowledge and expression,” AJ observed.
The Four Key Competencies of GenAI
This process creates a technology that is highly competent – at certain tasks. During his keynote, AJ enumerated what he found to be the core competencies of LLMs:
Fluency: LLMs can read and write in English and other languages, often with better grammar than most of us.
Creativity: These tools can create truly novel connections and ideas, whether analogies, poetry, or entirely new concepts.
Knowledge: By filling in the blank in billions of sentences of training data, LLMs internalize all the knowledge contained in them.
Logical reasoning: In what is probably the most surprising emergent component, these tools can make inferences and connect the dots in ways few anticipated.
A Principled Approach to GenAI in Law
“GenAI is a powerful, transformative tool,” AJ concluded. “But as with any tool, it can be utilized well or poorly.”
At Everlaw, AJ explained, we are committed to using GenAI well, backed by our core company philosophy: build for the long term. That means acting with integrity and discipline in all that we do. “We don’t care about being first,” AJ reflected. “We care about being the best.”
That approach has guided Everlaw’s three core principles for deploying generative AI: control; privacy and security; and confidence.
Zero Data Retention in EverlawAI
To that end, Everlaw has been working to ensure the privacy of all user data when interacting with our generative AI tools. That’s been the goal, “since we first started using OpenAI as our LLM partner,” AJ stated. “I’m pleased to announce that any data passed from Everlaw to OpenAI has zero data retention.”
“Zero data retention means that when your data is sent to OpenAI, they can’t store that data. They use it to process the task at hand and then remove it.”
Building to AI’s Strengths
Everlaw’s approach of building for the long term also means implementing generative AI in the places where it will have the best, most reliable impact. “The most important thing we do is deeply understand the capabilities of LLMs,” AJ described, “and design features that, as much as possible, play to their strengths while avoiding their weaknesses.”
We understand that the law is different. “The stakes are high,” AJ continued, “and the questions tend to be precise.”
“With high stakes and high precision, you really are rolling the dice when you rely on an LLM’s embedded knowledge base. This is how hallucinations happen. And when they do, the consequences can be significant.”
“So we want to stay away from use cases that rely on an LLM’s embedded knowledge.”
That means focusing on the areas where LLMs perform best: fluency, creativity, and reasoning – but not factual knowledge.
“Everlaw Review Assistant produces competent summaries that one would expect from an experienced attorney, in less than 30 seconds.”
- Gordon Calhoun, Partner, Lewis Brisbois
To translate these areas into the product, we lean into tasks that require fluency and reasoning. For creativity, we gauge the creative input of the LLM according to the task: less creativity for tasks like summarization, more for those requiring it to connect the dots, such as drafting a statement of facts.
To avoid using embedded knowledge, we focus tasks on the four corners of the documents at hand, rather than relying on facts the LLM may have internalized during training.
But we know that these tools are still imperfect. “So we also think that another critical part of building confidence is being able to verify GenAI’s work yourself.”
“We think of it as a really smart intern who can get work done incredibly quickly. You want to verify that work and make it your own. So in our product design, we want to make that verification really easy for you to do.”
That means having AI cite specific, immediately verifiable passages of text, page ranges, or Bates numbers to support its assertions. That way, you can check the work yourself, quickly and easily, and be confident in the outputs.
Doubling the Speed of Core Legal Work
The impact of these approaches is already emerging. Everlaw launched EverlawAI Assistant this July and AJ was joined on stage by one of the tool’s earliest adopters, Gordon Calhoun, a partner at Lewis Brisbois and a longtime leader in the ediscovery space. In piloting EverlawAI Assistant, Lewis Brisbois saw immediate impacts on core legal work, reducing the time needed for critical tasks – such as summarizing key documents and preparing for depositions, mediations, dispositive motions and trials – by at least 50%.
These tools “blow off the doors in terms of delivering a better, deeper understanding of complex, voluminous information to our legal teams in a time frame orders of magnitude shorter than ever before,” Gordon said. “Instead of an attorney taking 15 minutes to read and summarize the contents of a 50-page Word document in Notes or scour a 100-slide PowerPoint to identify the content needed to build your case, the Everlaw Review Assistant produces competent summaries that one would expect from an experienced attorney in less than 30 seconds.”
With impacts like this, it’s no wonder that the legal profession has responded with such urgency to the advent of generative AI tools.
But, as AJ showed in his keynote, “This isn’t magic. It’s math.” And those who understand the technology will, we hope, feel more empowered to talk about it to their peers, to clients, and even to the courts. And, ultimately, to leverage it in their own matters and to see results like those of Lewis Brisbois.
“Everyone that has an AI steering committee,” AJ concluded, “we’d love to meet with you.”
Everlaw AI Assistant Beta
See the future of AI in law. Apply to join the beta today.
Get hands on with EverlawAI Assistant and see the future of AI in law. Apply to join the beta today.
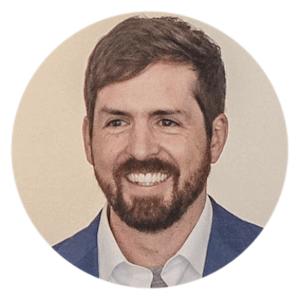
Casey Sullivan is an attorney and writer based out of San Francisco, where he leads Everlaw’s content team. His writing on ediscovery and litigation has been read by thousands and cited by federal courts.