Am Law 100 Firm Slashed Doc Review Time by Two-Thirds With GenAI
EverlawAI Assistant Coding Suggestions vastly simplified workflows
by Petra Pasternak
Takeaways
Using generative AI to review nearly 130,000 documents in a government investigation, the firm reported impacts including:
Reduction of review time by 50% to 67%, with one quarter of the personnel
Accuracy rates of 90% or higher, with performance at or above benchmark metrics for first-level attorney reviewers across recall and precision
Application of codes across 126,000+ documents in under 24 hours, following initial testing and validation
Increased consistency in coding decisions compared with first-level human reviewers
A more simple discovery workflow overall
Challenge
In the fall of 2024, a three-attorney team at a leading Am Law 100 firm was tasked with reviewing 126,000 documents for production in a large-scale government investigation related to a potential civil litigation matter. Their time frame was short and their budget limited.
With nearly 1,000 business and legal professionals providing a full range of legal and advocacy services from offices in the US and globally, the firm prioritizes innovation to deliver top-quality client services. To that end, its litigation team employed EverlawAI Assistant Coding Suggestions, a new large language model-powered ediscovery technology designed to accelerate the document review process.
Partnering with litigation managed services provider Right Discovery, they developed workflows, validation, and review procedures that apply the reasoning capabilities of generative AI at scale.
Results
The firm found that Coding Suggestions, which expedites the coding phase of ediscovery, delivered high-quality and consistent results in a fraction of the time it would have taken using traditional review methods.
“Being able to dive in and use generative AI technology in discovery is an opportunity to demonstrate clear value to our clients,” said the lead attorney on the matter. “Coding Suggestions saved us both time and money without sacrificing work quality.”
With Coding Suggestions, the team was able to make timely productions of relevant documents to the government with a quarter of the personnel needed in managed review for a comparable matter – and in less than half the time required.
After iterating and refining the coding criteria against which Coding Suggestions would analyze documents, the team was able to code 126,000 documents in about one day.
“EverlawAI Assistant marks a major step forward in document review, enabling future-looking legal teams to manage the explosion of data involved in litigation and investigations while significantly reducing manual review,” said Right Discovery CEO Kevin Clark.
How Coding Suggestions Drives Significant Savings
Document review is by far the most time-consuming and expensive component of litigation, with ediscovery costs making up nearly 80% of total litigation spend.
EverlawAI Assistant Coding Suggestions automates the coding phase of ediscovery by analyzing thousands of documents against instructions and criteria provided by users in natural language.
These criteria should cover descriptions of the case, the corresponding coding category, and the individual codes. Once Coding Suggestions has been instructed on the context of the case and goals of the review, the model then rates the documents as Yes, Soft Yes, No, and Soft No, and provides a rationale for each suggestion.
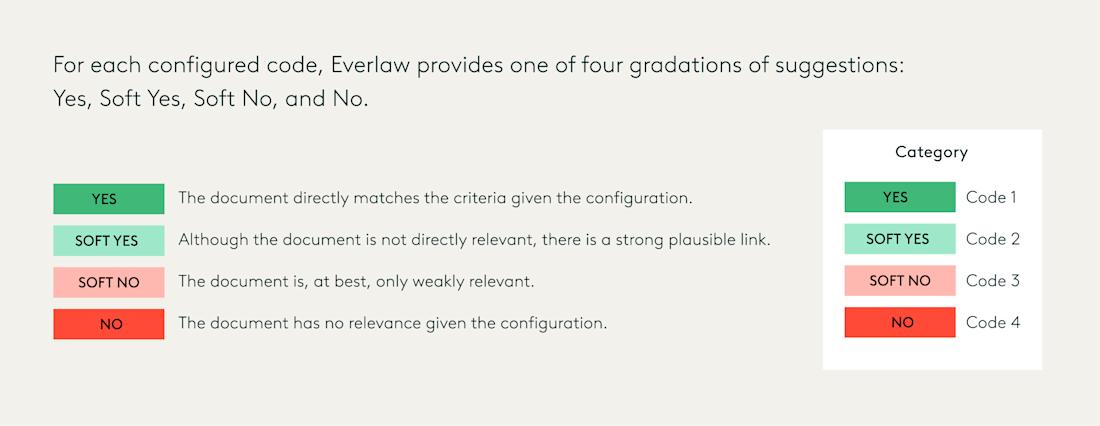
The firm attorney said this classification system was particularly impactful. When the Yes and No suggestions achieved a high accuracy level, the case team could then prioritize just the documents in the Soft Yes and Soft No categories for human review.
“The four-tier classification system simplified review immensely,” the attorney said. “It gave us clear document sets to prioritize for our teams to put eyes on.”
Establishing a New Workflow with GenAI
The firm decided to use Coding Suggestions as part of the review process in this complex case because of the high volume of records that would need to be sorted and categorized for a variety of search terms, categories, and issues.
“We had a sense that the vast majority of the documents would fall into the highly responsive category and we wanted consistency across those sets,” the lawyer said.
The lion’s share of the data consisted of work records – such as emails, email attachments, and MS Teams messages – that needed to be coded for responsiveness and tagged according to multiple requests, including nearly two dozen different issue codes.
“A human reviewer would take an immense amount of time making decisions on so many different data points. That’s an arduous task,” the lawyer said. “The AI basically solved that problem.”
Prompting Coding Suggestions for Best Results
To achieve the best results, review tools built on large language model technology require a set of nuanced instructions – known as prompts – at the start of the review process. Because the performance of Coding Suggestions is primarily determined by the quality of the prompts, an important part of the workflow is prompt iteration.
Case attorneys at the firm, with support from Right Discovery, created and honed the prompts for the matter in three main stages:
Stage 1: Creating the initial code criteria specific to the matter and describing the background and context at the code, category, and case level.
Stage 2: Selecting three subsets of records to iterate the code criteria against.
Stage 3: Running Coding Suggestions at scale across the entire 126,000-document set once it returned the desired results.
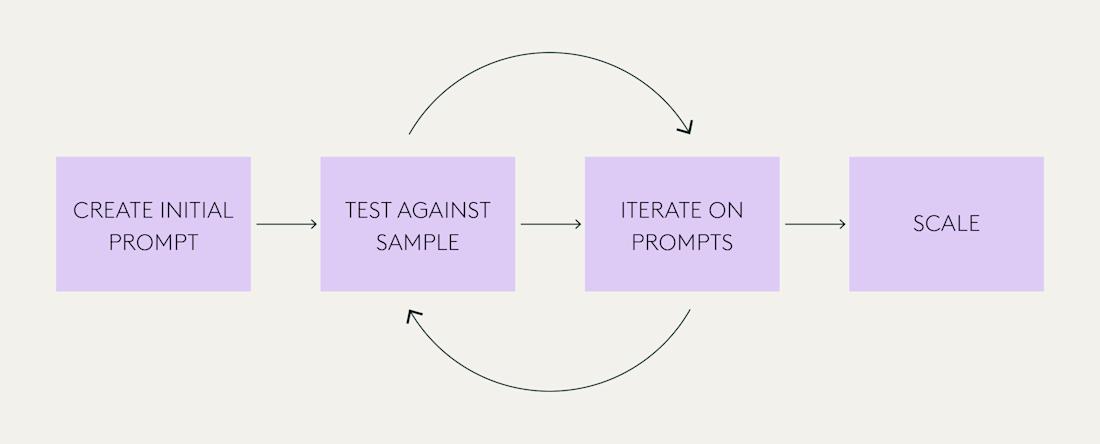
Each time a suggestion came back, the legal team evaluated it for quality, asking, for example:
Did Coding Suggestions categorize each document the way the attorneys would?
Was the tool being over or under-inclusive?
When Coding Suggestions went too far in any direction, the team edited the prompt to emphasize a particular point.
Right Discovery’s Kevin Clark said that ensuring the approach was accurate and defensible was paramount. Knowing GenAI can be validated with the same metrics as other AI tools, such as technology assisted review (also known as TAR), Right Discovery worked with the firm to determine the precision, recall, and F1 scores across the sample test set of documents before applying Coding Suggestions to the full review set.
Precision measures how many documents are actually relevant out of the number suggested as relevant; recall measures how many of the relevant documents are predicted to be relevant; and F1 is a weighted average of precision and recall scores that underlines a model’s overall performance.
Separately, when Coding Suggestions achieves a high accuracy level at Yes and No of the four gradations, legal professionals can prioritize those documents categorized in the middle, as Soft Yes and Soft No, for human review.
“The process of prompting Coding Suggestions is more organic than when you’re devising complex Boolean search terms."
According to Right Discovery and the firm, Coding Suggestions achieved a precision score of 0.63, a recall score of 0.85, and an F1 score of 0.72 for overall responsiveness in that final test set. These results are comparable to Coding Suggestions’ performance results in a series of earlier tests on real-world matters.
By the time the final test ran its course, Coding Suggestions was categorizing documents with 90% accuracy or higher, Clark said, matching or outperforming first-level attorney reviewers.
Prompting GenAI Takes Less Technical Skill than the Traditional Approach
The firm estimated that, in total, it took about 15 hours to tailor the optimal prompts for Coding Suggestions to accurately recommend codes for the vast majority of documents (including a learning curve with the new technology).
It was far easier to prompt Coding Suggestions using natural language than more traditional search and filter methods, the attorney said. And it eliminated the educated guesswork needed to identify the search terms that would help code for so many different data points.
“The process of prompting Coding Suggestions is more organic than when you’re devising complex Boolean search terms,” the lawyer said. “Working with the GenAI tech is much more conversational, and frankly, less technical than traditional tools.”
When inconsistencies did crop up between the attorney's coding decision and the way the AI coded a document, the discrepancies were at the very margins – and not highly relevant to the case.
“The document might be responsive to the document requests in question,” the attorney explained, “but only someone like me who had been intimately involved in the case details and perhaps even conversations with the government would know the document is, in reality, so low-value that it would be nonresponsive. I would feel comfortable coding that distinction.”
With that confidence, which came early in the process, the case team was ready to run Coding Suggestions on the full data set.
“Coding Suggestions simplified our workflow immensely and saved us a ton of time.”
Saving up to a Month of Attorney Review Time with GenAI
The firm estimated that a traditional managed review for 126,000 documents in the matter would have taken about 20 contract attorneys approximately four weeks to review and code.
With Coding Suggestions, just five team members were able to handle the work: three attorneys and the firm's director of litigation practice solutions, with support from a project manager at Right Discovery.
Once the model had all the necessary context about the case and review goals, the firm and Right Discovery were able to get through 126,000 documents in about one day.
“Coding Suggestions exceeded our expectations, showing more consistency in its performance than we would see from first-level human reviewers,” the lead attorney said. “It simplified our workflow immensely and saved us a ton of time.”
Visibility into Coding Suggestions’ Reasoning Boosts Confidence in Results
The firm’s director of litigation practice solutions said the ability to see how Coding Suggestions arrived at its recommendation was another key strength of the tool.
Coding Suggestions describes its rationale for determining document relevance in real time. That transparency made evaluation of the results of each prompt iteration easier and more efficient. Reviewing the Soft Yes and Soft No suggestions allows attorneys to quickly assess relevance, he said, which enhances review speed and accuracy while also reducing costs.
“Getting that feedback right away by just looking at the document is invaluable,” he said. “It really builds your confidence in the results and speeds up the review process.”
Once Instructed, Coding Suggestions Pays Dividends in Future Case Work
One of the key advantages of Coding Suggestions was its consistency, especially compared to human reviewers, the team found.
Where two different attorneys might interpret a document differently, Coding Suggestions read similar documents or identical forms of each document the same way.
This is especially important for maintaining a high level of work quality and avoiding issues later in the case when new requests for productions can become a point of contention.
“Government investigations can go dormant for 12 months and then we’re asked to produce a whole slew of new information,” the attorney said. “Coding Suggestions doesn’t just save time today, the benefits extend into the future.”
Coding Suggestions are a more reliable way of refreshing a case team’s memories months down the road because they are based on attorney-drafted prompts reviewed carefully at the time.
Compare that to reviewing individual coding on any random document; the future attorney picking the material up cannot be certain what was going on in the mind of the document reviewer. Having the coding prompts and decision rationales provides a much more uniform base from which to operate in such a situation.
Similarly, the valuable hours spent iterating on coding prompts at the outset could be recycled – or, if possible, given new lines of inquiry – used as a model from which to build on to address new requests.
It also makes quality control much easier and more effective.
“All things being equal, there are a number of reasons why the AI tool returned superior results to manual document review at this scale, and the consistency is by far the biggest,” the attorney said.
Staying Ahead of the Competition with GenAI Technology
By using Coding Suggestions, the firm was able to overcome the difficulty of quickly sorting and coding nearly 130,000 documents for highly nuanced issues and requests. Its consistency, accuracy, and speed significantly reduced the manual workload for the legal team, particularly during quality control.
“Coding Suggestions eliminates the guesswork involved in finding the most impactful search terms. It is much simpler to provide AI with macro-level instructions and then review the results,” the attorney said. “The quality control stage is significantly easier and less time-consuming due to the scale and consistency of Coding Suggestions.”
He emphasized the importance of staying ahead of the curve by working with emerging technologies like Coding Suggestions.
“The sooner we master these tools, the more value we add for clients, as they save time and money while maintaining or even increasing quality.”

Petra is a writer and editor focused on the ways that technology makes the work of legal professionals better and more productive. Before Everlaw, Petra covered the business of law as a reporter for ALM and worked for two Am Law 100 firms.