How to Interpret Predictive Coding Metrics
by Everlaw
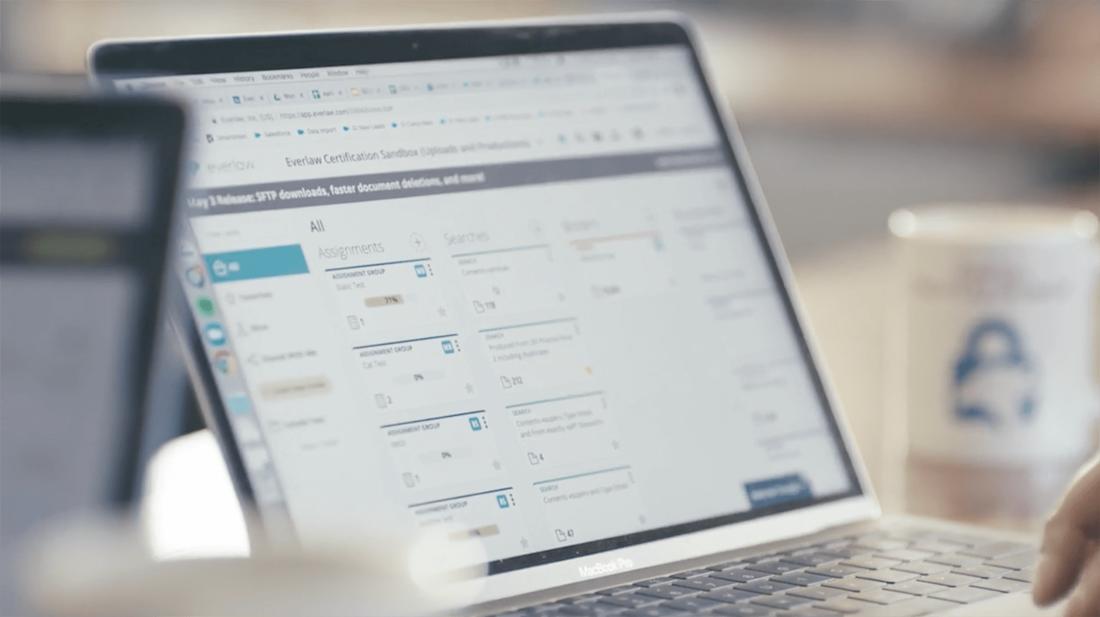
Ever wondered what all of the words used to describe predictive coding mean? It can be tough to remember the difference between precision and F1 – and to then use the definitions to improve the efficacy of document review. Below, we try to demystify these concepts, so you can strategically apply them on your case.If you need a refresher on how to set up a predictive coding model in the first place, start here. Once your model contains sufficient data, you will see the following analytics:
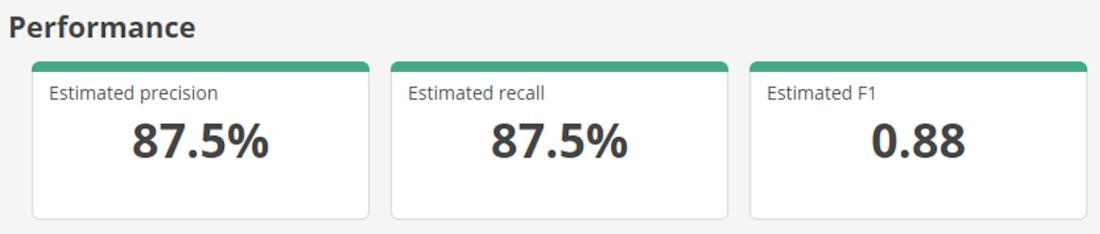
1) Estimated Precision
Precision is a measure of how well predictions match reality. To calculate it, we compare the proportion of documents you deem to be actually relevant to the documents the algorithm classifies as being relevant. Put another way, if the tool suggests a document is relevant, how likely are you to agree?
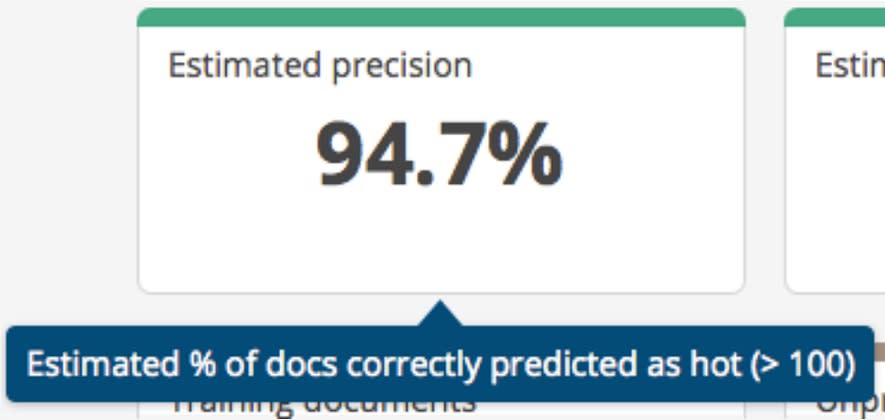
Here’s an analogy: imagine you had a database of all of the birds in the world, and you wanted to find all the flamingos. If your algorithm is trained to find all pink, long-legged South American birds, most of the results would, in fact, be flamingos. However, some of them might not be; some might be Roseate Spoonbills, which also fit that description.
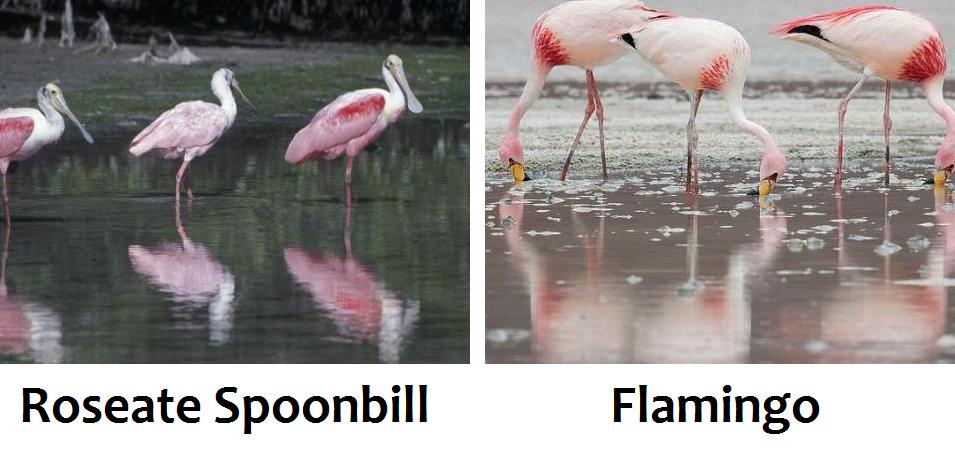
When a model has low precision, it means that there are files in the “likely hot” batch that you would actually rate as warm (or even cold!). These are sometimes known as false positives.One way to help improve the precision of your mode is increasing what the tool knows about the types of documents you have already rated, so it can better differentiate “somewhat relevant” from “very relevant.” In our flamingo example, this would mean adding details – like how many eggs are laid per “clutch” or how big the wingspan is – to weed out the spoonbills.In your case, you can remedy this by reviewing more documents in the high coverage portion of your “Training Set” graph.
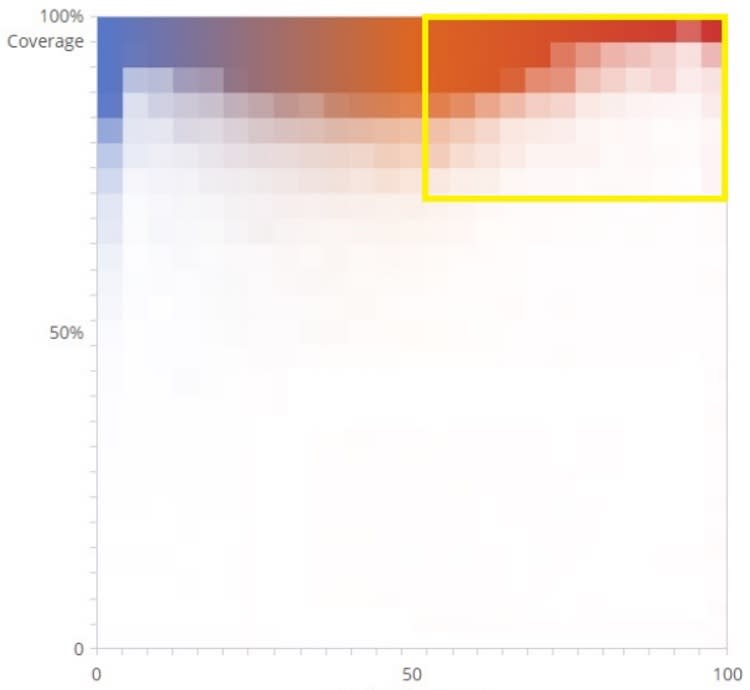
2) Estimated Recall
Recall is a measure of how comprehensive the predictions are. To calculate it, we compare where the actually relevant documents are found: the proportion of them in the set predicted to be relevant versus the total number of them in the case as a whole. Put another way, for any pertinent document, how likely is it that the algorithm would have predicted that it would be relevant? Or, how likely is it that there’s a super relevant document floating around out there that the algorithm isn’t aware of?
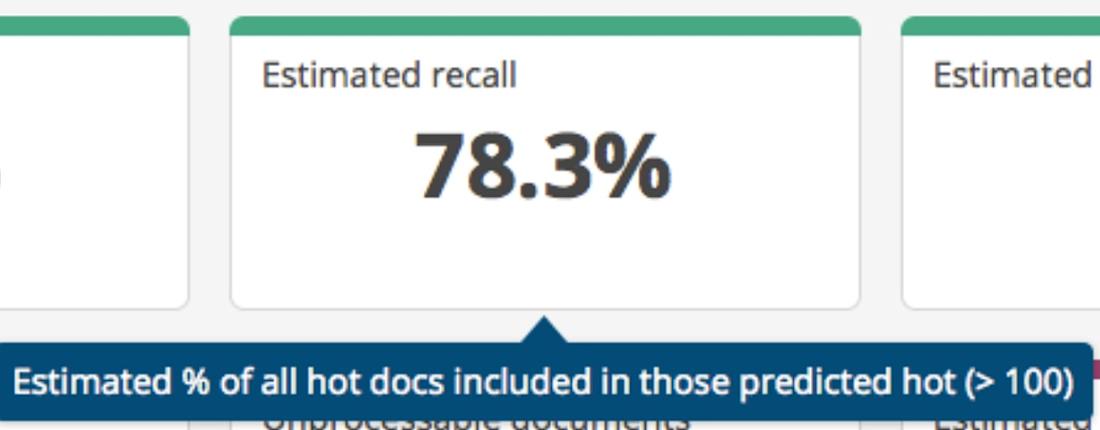
When a model has low recall, that means there are files missing from the “likely hot” batch that you would rate as hot. These are sometimes known as false negatives.One way to help improve the recall of your model is increasing what the tool knows about the types of documents you haven’t yet rated, so it can better find all the relevant ones. In our flamingo example, recall measures how many of the total flamingos in the world we’ve found. For example, we defined flamingos as being pink, but it turns out that there are also blue and orange flamingos too! Our algorithm hasn’t been looking for them, so it doesn’t have a good picture of all of the flamingos in the world.
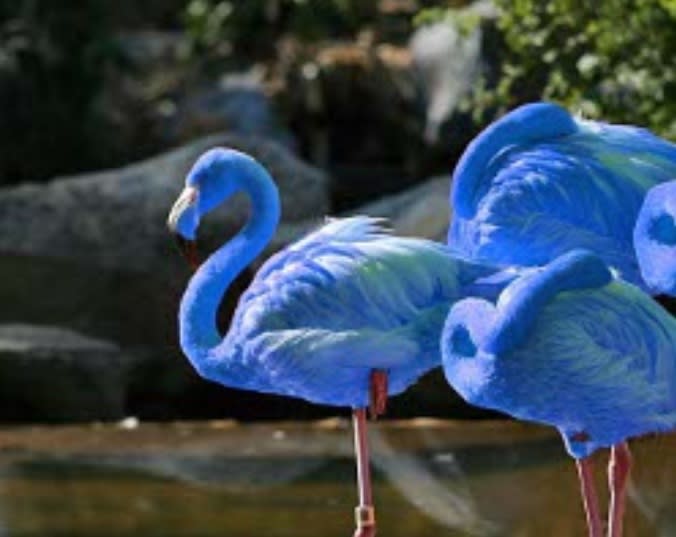
In your case, you can remedy this by reviewing more documents in the low coverage of your Training Set graph.
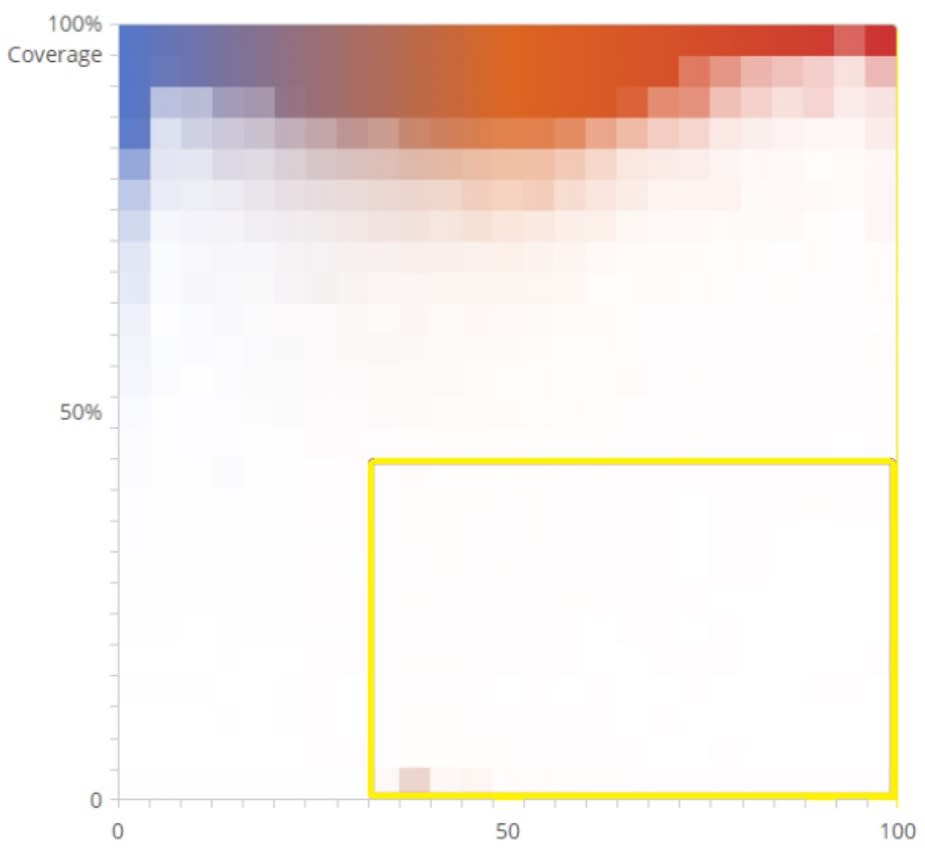
3) F1
F1 is a composite measure of a predictive coding model’s accuracy. It takes into account both the estimated precision and recall scores. Its possible values range from 0-1 (inclusive), with higher scores indicating more accurate models. There’s a natural trade-off between precision and recall – the more documents you review, the higher fraction of hot documents you’ll find (higher recall), but at the cost of seeing more false positives (lower precision). F1 scores often provide the best way to judge how well they have been balanced.
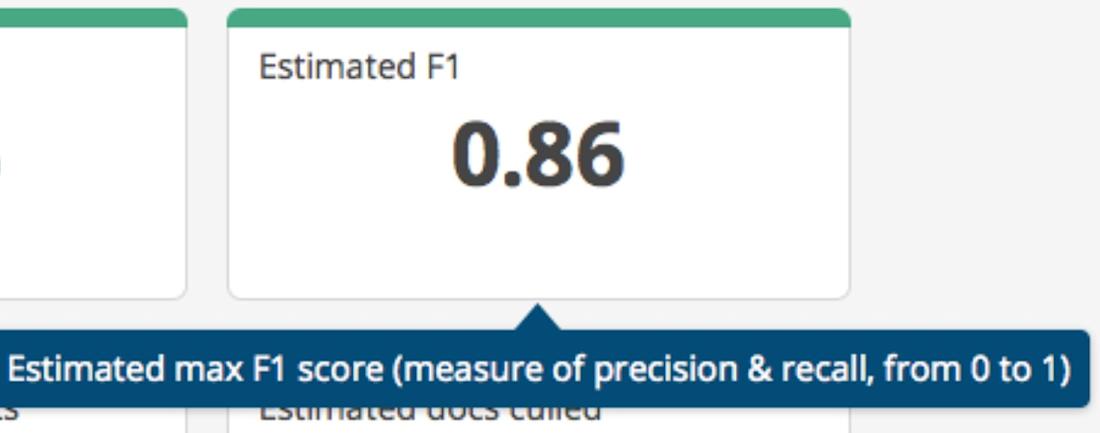
In our flamingo example, that means evaluating how close you’ve come to finding all the flamingos in the world. On the one hand, you may not have the time to list every country in which they might be found or every possible wingspan. (Or, in legal terms, you need proportionality.) On the other hand, you don’t want to end up with a list that only has half of the flamingos in the world, or any conclusions you draw might not be right. (Or, in legal terms, lacking evidence to make your case.) F1 is a measure of how well you can balance those competing demands.Hopefully, understanding these terms can help you find the happy medium in your case. Or, the right number of flamingos!
For more information on predictive coding and resources on setting it up for your case, read this guide.

Everlaw’s advanced technology empowers organizations to navigate the increasingly complex ediscovery landscape, tackle the most pressing technological challenges, and chart a straighter path to the truth—transforming their approach to discovery, investigations, and litigation in the process.