Everlaw Clustering: 3 Use Cases To Boost Efficiency During Review
by Kanika Priyadarshi
Until recently, discovery in ediscovery has often been associated with search. Legal teams are typically forced to search for critical and relevant information in ever-growing data sets with no obvious place to start. And the most challenging aspect of the process can be just knowing where to begin, leaving reviewers to rely on instinct, craft complex searches, and sometimes even use machine learning tools to find pattern trails to follow.
Review tools have gone through an evolution over the last few years. The most effective solutions feature intuitive user interfaces for discovery, time-saving capabilities, like Clustering, that can reveal the hidden insights of documents at scale, and tools that enable automated workflows that enhance and expedite the review process.
What Is Clustering?
Clustering is a machine learning tool that helps detect trends within data sets. The tool visualizes and organizes documents within a given set that are conceptually similar – and it does this without requiring users to train the model. Instead, Clustering uses an unsupervised machine learning algorithm that identifies and analyzes metadata (e.g., author, subject, title, and email sender/recipient) and text across an entire data set to determine conceptual similarity among digital files and documents. Clustering also utilizes what is known as a density-based Clustering algorithm, which allows users to visualize trends within data sets more easily than with traditional K-means Clustering algorithms.
How Clustering Works
Clustering visualizes documents in your dataset by conceptual similarity. As a result, it generates insights about concepts in your documents without requiring any user input (e.g., building a search). Documents can be effortlessly filtered and sorted, empowering you to explore the dataset to discover new search terms, find relevant documents, and make critical decisions early on when it comes to prioritizing and organizing documents for review. Clustering can also be used to quickly find and eliminate irrelevant documents (such as junk email) and filter them away, effectively reducing the billable size of projects.
Clustering aids in post-review QC and tracks down concepts in key documents. With the ability to scale to millions of documents, Clustering enables targeted review, saving legal teams time and cost. Although traditional search tools require users to have a baseline understanding of what is in your documents and what to search for, Clustering enables users to learn about data without any prior background, making it an extremely valuable tool during early case assessment and other critical workflows throughout the discovery process.
Clustering Use Cases
Simply put, legal professionals can leverage Clustering to get things done reliably, on time, and without introducing timeline uncertainties for client requests. In particular, there are three specific Clustering use cases during the review stage, when utilized properly, that can have a tremendous impact:
Clustering Use Cases: Early Case Assessment
Spotting and identifying which documents and files are relevant to a particular case can help establish the timeline of events and lay the foundation for the legal strategy. But often, there are mountains of data (duplicate documents and redundant information) that can lengthen the initial review. Ediscovery software that utilizes automation technology and machine learning can expedite this process.
For example, Everlaw Clustering uses unsupervised machine learning to quickly pinpoint conceptually similar files and presents them via an intuitive graphic display, making it easier to uncover valuable insights into your data set without manually building a search during Early Case Assessment.
Also, documents can be easily filtered and sorted, enabling Everlaw users to explore their data set in order to find documents that potentially have evidentiary value, discover new search terms, and make critical decisions early on (i.e., prioritizing and organizing documents for review).
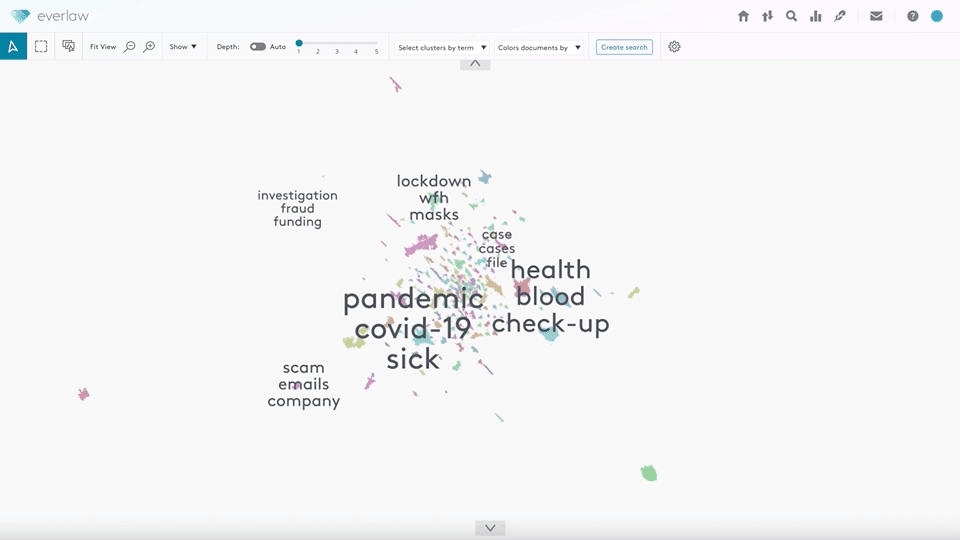
Clustering Use Cases: Review and Investigations
Clustering can be very useful to assist team members during data review and investigations to identify meaningful concepts. For example, users can leverage Everlaw Clustering capabilities to group related unlabeled documents together, and then use predictive coding to identify those groups of documents that are highly predicted to be relevant. This pairing is particularly beneficial when extracting digital files and documents within large data sets.
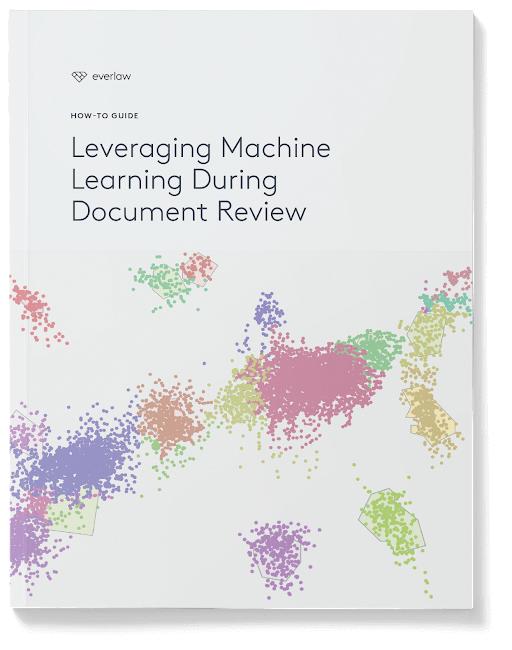
Clustering Use Cases: Quality Control
People make mistakes, and so do legal professionals. That’s why conducting quality control is a vital step during the review process. For example, an attorney responsible for assessing the quality of review decisions made by their team needs to make sure nothing slipped through the cracks. That means double-checking that all relevant documents were reviewed, coded, and rated correctly. Ensuring that all documents are categorized accurately, especially before the production is exported, can reduce the risk that privileged, confidential, or simply nonresponsive information is produced.
Documents can be clustered based on critical subject matter to verify coding decisions and ensure that relevant documents in hot clusters were identified during the review. For example, with Everlaw, you can utilize the coding overlay to identify potentially uncoded or incorrectly coded documents.
Open a New World of Ediscovery Insights with Everlaw Clustering
Everlaw Clustering is seamlessly integrated with the Everlaw platform to help legal teams accelerate finding key pieces of evidence, mitigate the risk of human error, and confidently navigate ediscovery at terabyte scale. Clustering also complements Everlaw Predictive Coding’s supervised learning for more powerful AI workflows.
More specifically, here’s what Everlaw Clustering enables legal teams to do:
See clusters dynamically separate and merge based on zoom level through dynamic zoom.
Overlay predictive coding models and use the prediction scores to find hot documents.
Overlay ratings and codes to prioritize certain document sets or validate review decisions.
Recluster at any given moment.
View the most common terms found in clusters and any transcribed A/V files.
Filter visualization to only display a specific search.
Access similar documents in a cluster through the context panel in the document review window.
Open documents directly into Data Visualizer.
To learn more about Everlaw Clustering Use Cases and Predictive Coding technology, check out our latest eBook, “Leveraging Machine Learning During Document Review: A How-To Guide.”
Kanika Priyadarshi is a Product Marketing Manager at Everlaw focusing on marketing and go-to-market strategies for AI, cloud adoption, big law, and mid-market law firms.