The Use of AI To Underpin Technology Assisted Review in the UK
by Gregory Campbell
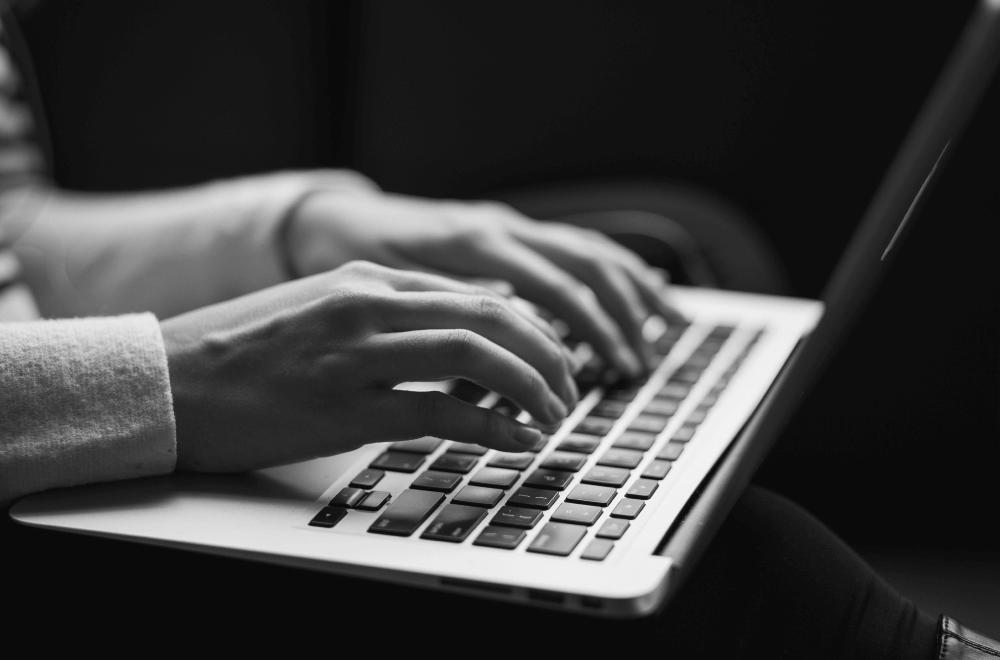
There is a long-running perception that any countries outside of the US are somewhat of a backwater when it comes to the use of technology in the legal sphere. Whilst this may have been true previously, in my experience of speaking to organisations leveraging technology for these purposes, the UK has certainly caught up considerably over recent years.
The concept of Artificial, or Augmented, Intelligence (AI) is not, in my view, something that can easily be defined precisely, and there is much debate around what constitutes this. It is easy to get caught up in feature-functionality comparisons, to be overwhelmed by fear, uncertainty, and doubt (or FUD) and to be confused by what seem to be conflicting explanations and definitions. As an individual who has worked not only as a legal practitioner, but also in the ediscovery service industry, a litigation support team, and for several technology companies, I have reached one conclusion; technology is but a path to the outcome.
With this in mind, whilst the use of tried and tested Technology Assisted Review (TAR) processes, in particular the machine learning algorithms that power predictive coding and clustering, have become more widespread, the availability of AI-focussed technology that pulls together these technologies and provides a consumer-grade user experience underpinned by enterprise-grade functionality has been somewhat lacking until comparatively recently.
Primarily through my journey with Everlaw, I have been fortunate to witness first-hand how organisations are now more willing to move from legacy tools to modern cloud-native solutions, to be able to experience better ways to achieve the outcomes they need, with a focus on solutions as opposed to individual feature-functionalities.
Civil Case Law and AI in England and Wales
In respect of the use of AI technology in the scope of civil litigation in England and Wales, there have been several decisions in respect of the use of what can be seen as one of the building blocks of AI in the legal sphere, namely predictive coding, most notably in 2016. The earliest of these 2016 judgments approved for the first time the usage of predictive coding in matters where both parties consented (Pyrrho Investments Ltd v MWB Property Ltd [2016] EWHC 256 (Ch).
The second, and later, of these judgments also permitted the usage of predictive coding in a matter by one party, notwithstanding the other party’s objection to using it (Brown v BCA Trading Ltd [2016] EWHC 1464 (Ch)).
The use of predictive coding to establish relevance has also recently been confirmed as “an appropriate way to proceed” in the high court (Isbilen v Turk & Ors [2022] EWHC 697 (Ch)).
The Disclosure Pilot Scheme: Reducing the Burden of Disclosure With AI
The Disclosure Pilot, in the form of Practice Direction 51U (PD51U), has a central theme of using technology to reduce the burden of disclosure in those civil litigation matters in the Business and Property Courts in England and Wales where it applies, together with the obligation to cooperate around its use.
PD51U sets out both in its body (Paragraphs 3, 6A and 9), and in the Disclosure Review Document (DRD), that consideration must be given, for example, to the use of software or analytical tools, including workflow and coding strategies, technology or computer-assisted review (otherwise known as TAR/CAR), email threading, deduplication, near-duplicate identification, concept clustering and foreign language analysis, even before the Case Management Conference (CMC).
Whilst technologies including those listed above are specifically mentioned, the DRD, which is an important part of PD51U, does, however, make it clear that parties should not feel constrained from proposing new forms of technology which may be developed in the future, and which may be appropriate for use in any given case.
This certainly does not exclude the appropriate use of AI methodologies above and beyond more traditional Technology Assisted Review for suitable matters. Although discussing these in advance with the court and/or the other parties to any matter in which you propose to use them is likely to be considered sensible by your legal or consulting advisors.
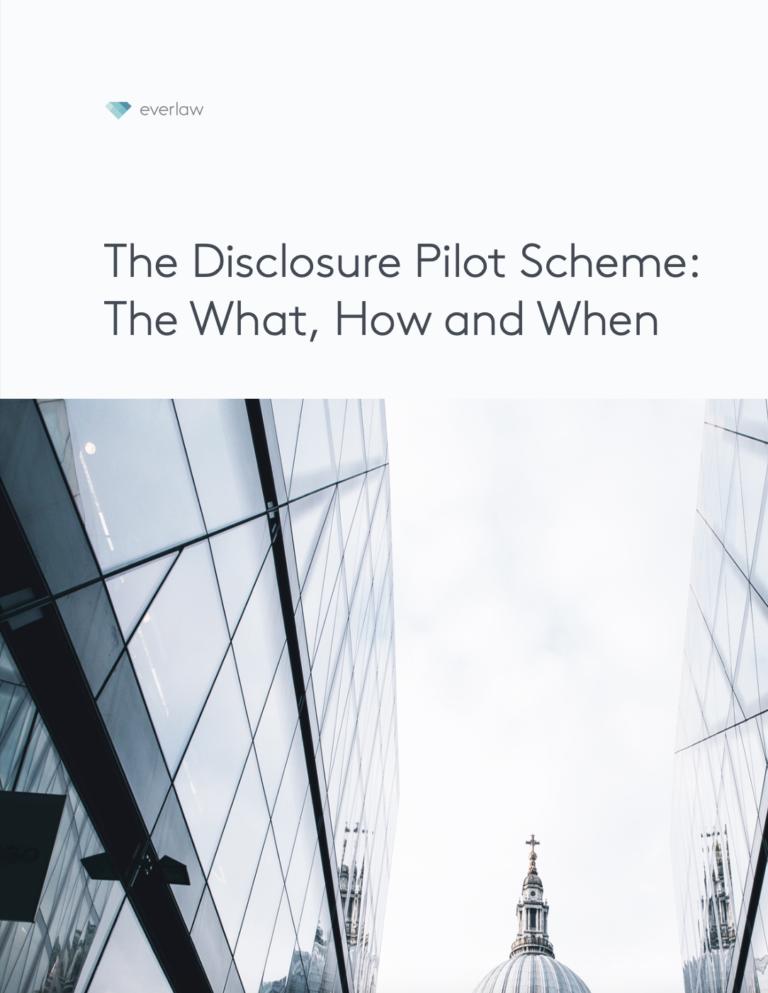
AI in Regulatory and Criminal Matters
When applying AI, including TAR tooling such as predictive coding or clustering beyond civil litigation, for example, in respect of regulatory matters, any use of technologies to reduce the burden of review and production is likely to be determined by legal or consulting advisors and is best discussed with the regulator in question before embarking on their usage.
Subject to this proviso, UK regulators are likely to entertain the controlled and considered use of any technology that can streamline and improve the process of providing them with evidence.
Additionally, in relation to criminal matters, the Crown Prosecution Service (CPS) Disclosure Manual for England & Wales, Chapter 30 – Digital Material, explicitly states that: “Technology that takes the form of search tools which use unambiguous calculations to perform problem-solving operations, such as algorithms or predictive coding, are an acceptable method of examining and reviewing material for disclosure purposes.”
This would seem to point towards those technologies such as predictive coding, which can more easily provide statistical defensibility. However, it would not necessarily preclude other uses of AI technologies, with appropriate safeguards in place. Although consultation with legal or consulting advisors, and potentially the receiving party, is likely to be sensible in any given situation.
AI and Technology Assisted Review in Everlaw
Everlaw supports the core AI-focussed TAR technologies identified above, in particular providing both supervised and unsupervised machine learning functionalities in the form of our predictive coding and document clustering modules, both of which are fully integrated into your Everlaw toolbox.
Our predictive coding and document clustering functionalities provide a consumer-grade user experience underpinned by enterprise-grade functionality. In summary:
Everlaw’s predictive coding is based on continuous active learning (CAL) and is as easy to use as other platform features, with a combination of intuitive graphical interfaces and wizard-driven setup processes. Everlaw learns continuously from reviewer decisions, enabling reviewers to use their standard workflow (assigning ratings and codes, attributes to reviewed documents, etc.) to teach the system how to find more documents that are likely to be responsive, or otherwise, on their behalf. Common use cases include the prioritisation of documents and the automation of quality control processes.
Everlaw’s document clustering can be useful at the beginning of your review. For example, for identifying key terms in unknown and less well-known datasets, and as the review progresses, it can be deployed for quality control activities, with users able to overlay the aforementioned predictive coding models over document clusters in a highly visual and intuitive manner. Through the use of these overlays, users can, in effect, combine supervised and unsupervised machine learning in a practical application of AI.
The Defensibility of AI-Focused Approaches
The key to any process in the legal sphere is its defensibility if challenged. The approach to the use of AI or Technology Assisted Review technologies in the UK is that this defensibility is more hinged on the methodology and process used to ensure proper use of the technology employed in the exercise, together with keeping adequate audit trails to provide for accountability in this regard, as opposed to any obligation to use any particular system or algorithm in any particular matter situation.
Certain tools and methodologies will suit different scenarios more closely than others, so, for example, attempting to use predictive coding for prioritisation in a document set that is entirely responsive may not produce good results (unless you refine your approach, potentially focussing your predictive coding models on issues that form a subset of that responsiveness).
A key way to help you to better reach your goals is, therefore, through proper planning and a focus on desired outcomes, in preference to over-reliance on technology alone.
Everlaw can support the defensibility of your use of machine learning technology in two key ways, both in respect of the tooling we have integrated around our predictive coding and also through the support of our Customer Success team.
Everlaw’s easy-to-use predictive coding toolset incorporates multiple fully integrated performance metrics. This includes easy-to-access results relating to precision, recall and F1, that are generated automatically. Should you require a higher threshold of defensibility, it is also possible to use holdout sets (either basic or rigorous), which act as controls to generate more accurate statistics. This is complemented by the ability to overlay your predictive coding models over the clustering model for quality control purposes, as set out above. Everlaw also automatically keeps audit trails of user activity, which can be filtered and exported at will.
On the people side of the equation, Everlaw’s Customer Experience team is well versed in our predictive coding and document clustering functionalities, and is available to assist your team in their usage as part of your Everlaw subscription. Should additional statistical information be required (elusion testing and confidence levels/intervals, etc.), this can also be calculated with or without the support of our Customer Experience team.
I hope the above thoughts have been useful, and I will finish where I started; technology is but a path to the outcome. That said, the use of better technology, in better ways can lead to better outcomes, so please get in touch if you would like to find out more about how Everlaw can potentially help you to move beyond feature-functionalities towards solutions that drive these better outcomes.
Are you interested in learning how to utilise machine learning during document review? Download a free copy of our eBook, “Leveraging Machine Learning During Document Review: A How-To Guide.”

Gregory Campbell is a Principal Solutions Architect for Everlaw in the UK, acting as an ediscovery subject matter expert. He is a practising solicitor, originally training with a Silver Circle London legal firm, and subsequently practising as a litigation and dispute resolution focussed solicitor in London.